Uncovering The Perils of General-Purpose AI: Why Domain Knowledge Matters
Learn about specialized AI agents, domain knowledge, and how they outperform general-purpose AI models to stay ahead of the AI curve.
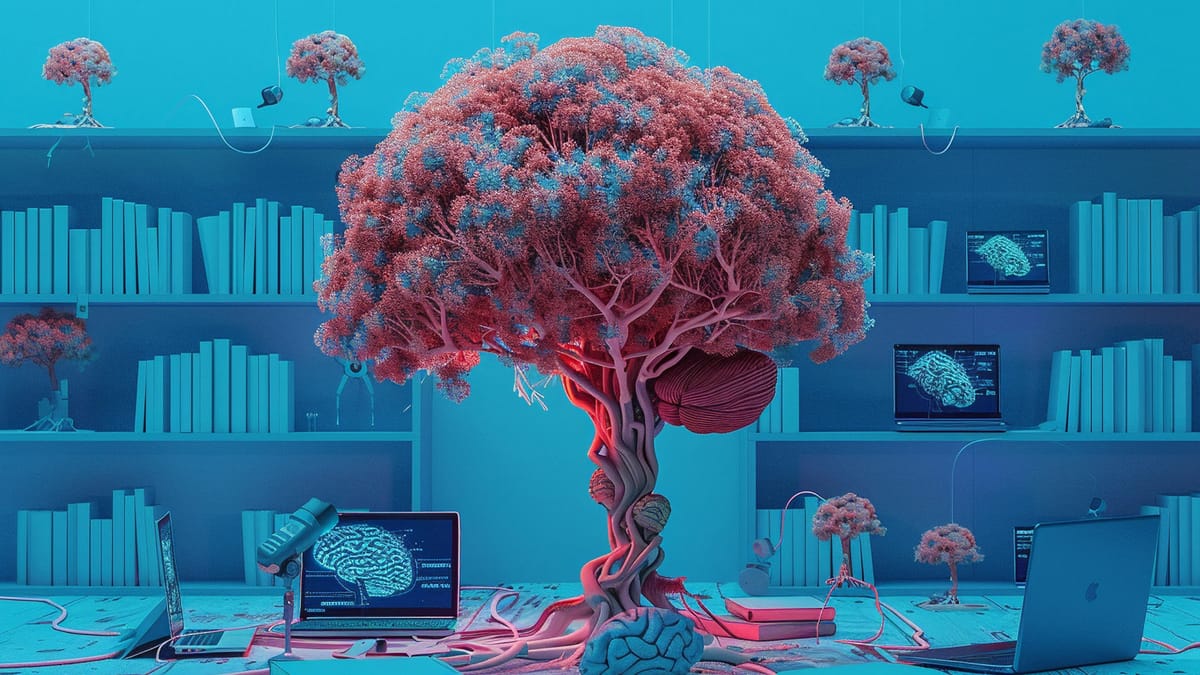
Artificial intelligence (AI) has captured the imagination of the world, with millions of users exploring the boundaries of what is possible every day. But the vast majority of existing AI systems face severe limitations, lacking the depth of knowledge necessary for operating in complex, cutting-edge, or personal domains.
Imagine asking a mechanic to fix your computer, or a chef to perform surgery. This is essentially what occurs when we interact with generalized AI tools. These "jack of all trades" AI systems are trained on broad subject matter, leading to a surface level understanding of knowledge domains which can be echoed and repeated, but nothing more.
The problem here is not the underlying technology itself, but its current pursuit: to be an all-encompassing solution that caters to everyone.
This ultimate goal from centralized players raises many concerns, including shallow expertise, rising costs, privacy risks, and more.
So what's the answer?
At Gaia, we are building the solution: Specialized AI trained on domain-specific knowledge bases.
In this piece, we'll dive deeper into why general-purpose AI models are ineffective for many use cases and explore the need for specialized AI.
General–Purpose AI Models Don’t Solve Problems
As mentioned earlier, general-purpose AI models aim to solve all problems — yet in their ambition, they solve none perfectly well.
Sam Altman, the CEO of OpenAI, talking about ChatGPT in December 2022.
To reference Sam Altman, ChatGPT and other popular AI models are simply ‘good enough’ at ‘some things’ to create a ‘misleading impression of greatness’. The inherent limitations around general-purpose AI systems make them unsuitable for any serious use cases where expert knowledge bases are required.
Diving deeper into the details, here are four limitations of general-purpose AI:
Broad competence and shallow expertise result in hallucinations
Generalist AI models often hallucinate when returning outputs, providing inaccurate or irrelevant information around specialized topics. The reason behind this related to the vast, generalized datasets these models are trained on. The broader a dataset, the more ill-equipped it is to handle the depth and nuance needed for specialized queries.
Similarly, domain-specific knowledge from subject-matter experts (like peer-reviewed papers in science journals, or case laws from judicial bodies) moves extremely fast, requiring consistent updates. It is a logistical nightmare to expect generalist AI models to keep up with the pace of new, emerging knowledge.
General-purpose AI models struggle with accessing frontier knowledge from subject-matter experts and fail to integrate that knowledge at the same pace.
Even in the face of these limitations, generalist AI models will still attempt to respond to queries by resorting to inaccurate hallucinations — which can be misleading at best, and mission-critical failure at worst.
Centralized AI models often inherit biases
Centralized AI models, in their pursuit of universal applicability, often fall prey to inherent biases inherited from their training data. The datasets fed into AI models embed these biases, which can result in skewed, unreliable, and even discriminatory outputs.
A well-known example is in political discourse:
Let’s see what happens when we ask a general-purpose AI about two US politicians and their recent public incidents. From the image above, we can see the responses are lukewarm and seemingly non-controversial.
The incident in the first question occurred on 13 July 2024, with the AI model refusing to answer, citing: “I don’t always have access to the most up-to-date information.”
But interestingly, the same AI model answers the following question with a citation about an incident that happened more than a week later — 21 July 2024.
This incident is not isolated to political biases. Studies and experiments have found popular AI models to be biased against race, disabilities, religion, and even language dialects.
Privacy and data management are not under your control
Privacy and data management are critical concerns when using centralized, general-purpose AI models.
Centralized AI models record, process, and store every data input from users, giving users zero visibility or control over this process. These systems are built and engineered within a black box. This means there’s no way for users to demand to see their data trail or revoke access to the data they entered into these models.
Enterprises in particular face significant risks, as sensitive data — ranging from trade secrets to financial information — can inadvertently be exposed to the world.
Contextual understanding is the need of the hour and is currently missing
General-purpose AI models excel at broad pattern recognition, helping them act as smart parrots that can quickly generate a response to user inputs. However, this isn't optimal for professional environments where grasping the context of industry-specific tasks is critical.
Technical jargon, regulations, contextual relationships, and other nuances are beyond the scope of general-purpose AI. This limits their utility, especially in fields like law, medicine, or engineering.
But can't we customize and fine-tune LLMs to specific use cases?
You can, but costs are prohibitive for most individuals and even small to mid-sized businesses.
Training a custom GPT-4 model can easily start at $2-3 million and also requires significant computational resources and infrastructure.
In short, generalist AI models fall short of being useful outside of simplistic and non-critical use cases.
Next, let’s explore the alternative — specialized AI trained on domain knowledge.
The Need for Domain Knowledge and Specialized AI
Specialized AI agents are trained on domain-specific knowledge, data, and algorithms. All of these inputs are then optimized to deliver contextually relevant and accurate results.
How does the training process actually work?
Here’s a high-level overview of how specialized AI agents work:
- Data collection: Specialized AI agents gather data from curated sources, such as industry reports, research papers, and proprietary datasets.
- Embedding knowledge: The agent uses embeddings to convert specialized concepts into vector representations for efficient querying and retrieval of information.
- Contextual understanding: The AI agent learns contextual relationships within the domain, enabling it to provide accurate and relevant outputs.
- Real-time inference: The agent applies this specialized knowledge to user queries in real-time, delivering actionable insights tailored to the specific field.
- Continuous learning: New data and updates are regularly incorporated into the model to ensure it stays current with evolving knowledge in the domain.
This process can be more involved than using a general-purpose AI model. But considering the many applications a specialized AI agent brings to the table, the rewards outweigh the effort. Gaia abstracts away the difficulties associated with building specialized AI agents and models for hyper-specific use cases.
Understanding Gaia and Specialized AI Agents In Depth
Gaia is a modular, decentralized AI infrastructure designed specifically to support the development and deployment of specialized AI models and agents. Individuals, businesses, and developers can use Gaia to create, fine-tune, and deploy AI agents that adapt and grow in real-time.
More importantly, Gaia is building a new economic model to incentivize knowledge creators and node operators contributing to AI, helping them monetize their expertise.
Now, we have learned the basics of specialized AI agents and how they work. And we also know about the perils of using general-purpose and centralized AI models.
In the next section, we will explain how specialized AI offers practical utility and applications for individuals and enterprises alike.
Deeper contextual understanding offers useful insights
Specialized AI agents can grasp and interpret knowledge at a deeper contextual layer catered to any use case. Personal agents can be built trained on specific historical references, cultural nuances, cultural norms, and individual sensitivities.
For businesses, this contextual understanding can involve industry jargon, research, regulations, and niche-specific risks. Unlike general-purpose models, these agents are tailored to their domain, offering insights that are valuable, actionable, and secure.
Ex: Harvey, a legal AI platform, is built on decades of excellence in law and it helps in drafting paperwork, legal and tax research, and more.
How does Gaia make this work?
Gaia’s decentralized AI infrastructure allows anyone to train specialized AI agents on domain-specific data across a distributed node network. Each node captures the intricate relationships between concepts using fine-tuned embeddings, which are then processed using realtime data updates.
This continuous learning approach ensures that each AI agent stays accurate and relevant, accessing the most up-to-date knowledge.
Ability to handle more complex, domain-specific tasks
Specialized agents can tackle complex tasks requiring domain expertise, like legal research, financial forecasting, or medical diagnoses — where precision is critical.
Extensive and curated datasets relevant to the field are used to train and fine-tune these models. With encoded rules and heuristics, these agents serve as experts on demand. Moreover, they can autonomously adapt to new data and regulations, ensuring that outputs stay accurate, updated, and compliant.
Here are a few examples:
- On-demand compliance support for OnwardAir, an aerospace company developing safe and affordable eVTOL aircraft.
- Financial advisor trained on high-quality data of Samsung’s investment arm — SamsungNext.
- NormAI is a regulation-based AI agent that helps businesses with autonomous compliance and risk management.
Fine-tuning and customization functionalities add precision and relevance
One important aspect of AI agents is the ability to fine-tune and customize them for any use case. This means that data can be curated for specialization, but also integrated into specific enterprise-level workflows to achieve more relevant outputs.
How does Gaia make this work?
Gaia provides a modular, open-source architecture that allows businesses to fine-tune and customize AI agents to specific workflows and domains. Using decentralized compute power and domain-specific data nodes, Gaia optimizes agents for targeted tasks, enabling continuous learning and optimization.
This approach ensures each AI agent adapts quickly to the unique needs of the business, resulting in more relevant and precise outputs.
Ex: ConverzAI acts as a virtual recruiter for businesses, improving their candidate experience and scaling the candidate engagement processes.
Better equipped to adhere to industry-specific regulations and standards
Specialized AI agents are uniquely suited to navigate and comply with industry-specific regulations and standards. Unlike general-purpose models, these agents are designed with a deep knowledge of regulatory frameworks, making them more effective in ensuring legal and ethical adherence.
Whether it’s healthcare data management under HIPAA or compliance with GDPR in Europe, specialized AI systems can be customized to meet these requirements.
How does this look in action?
- AI agents provide transparent decision trails that can be audited for compliance.
- Employ built-in safeguards and ethical checks for evaluation and internal audits.
- Set up automatic flagging for actions or documentation that might violate regulatory standards.
Ex: Florence, an AI-powered clinical co-pilot, developed by Anterior is HIPAA Compliant.
Evolution of data with domain-specific updates is easier
Keeping models updated with the latest domain-specific data is simpler with specialized AI. This agility in data absorption is particularly vital for enterprises looking to embed AI agents into their workflow.
Imagine this superpower in domains like,
- Medical research — where new studies and treatments emerge daily, or
- Finance — where market conditions and regulations rapidly change
Specialized AI can swiftly integrate these updates, ensuring that insights and recommendations always reflect the latest industry developments.
How Gaia makes this work?
Gaia’s decentralized knowledge nodes continuously gather and verify domain-specific data from pre-defined sources across the network. The AI agents undergo incremental learning, with new data integrated without the need for full retraining, ensuring seamless updates.
Moreover, Gaia enables data tracking and version control, allowing updates to be rolled back if necessary to maintain data integrity.
Gaia: Making Specialized AI Agents Accessible
Specialized AI agents that incorporate domain-specific knowledge will lead the future of AI — there’s no doubt here. But to make AI inclusive and user-centric, our next aim should be around improving access to decentralized, specialized agents to avoid the threat of centralization.
Gaia is pioneering this transformation by providing the essential decentralized infrastructure for building and deploying advanced AI agents. With its open-source, modular framework, Gaia empowers developers to fine-tune AI agents for specific use cases and embed them within their tech stack or workflows.
Our ultimate vision is to align the incentives behind AI to be more democratic and fair to knowledge creators and contributors.
What next? Explore Gaia and learn more about the platform:
- Use and interact with all of Gaia’s live agents
- See all the large-language models that Gaia supports.
- Learn how to run a Gaia node
- Secure a Gaia domain name for yourself
- Read and learn more about Gaia using our documentation